AI Advancements: OpenAI and Anthropic’s Collaboration with Federal Authorities
Introduction
In a significant move towards ensuring the responsible development of artificial intelligence (AI), OpenAI and Anthropic have agreed to provide federal authorities with early access to their latest AI models. This initiative is designed to align AI development with regulatory standards and ethical guidelines, fostering a safer and more transparent AI landscape.
The ‘Strawberry’ AI Model
The centerpiece of this collaboration is a new AI model codenamed ‘Strawberry.’ This model is reported to have enhanced reasoning capabilities, making it a notable advancement in the field of AI. The improved reasoning abilities of ‘Strawberry’ are expected to have wide-ranging applications across various sectors.
Applications and Implications
- Natural Language Processing (NLP): The ‘Strawberry’ model’s advanced reasoning capabilities can significantly enhance NLP tasks. This includes better understanding and generation of human language, which can improve virtual assistants, chatbots, and translation services.
- Decision-Making Systems: Enhanced reasoning allows for more sophisticated decision-making systems. These systems can be applied in areas such as autonomous vehicles, where making real-time, accurate decisions is crucial.
- Healthcare: In healthcare, AI models like ‘Strawberry’ can assist in diagnosing diseases, personalizing treatment plans, and managing patient data more effectively. Improved reasoning can lead to more accurate predictions and better patient outcomes.
- Finance: The financial sector can benefit from AI advancements through improved fraud detection, risk assessment, and automated trading systems. Enhanced reasoning capabilities can lead to more reliable financial models and predictions.
- Education: AI can revolutionize education by providing personalized learning experiences, automating administrative tasks, and offering intelligent tutoring systems. The ‘Strawberry’ model’s capabilities can enhance these applications, making education more accessible and effective.
Regulatory and Ethical Considerations
By giving federal authorities early access to their AI models, OpenAI and Anthropic aim to ensure that these technologies are developed responsibly. This collaboration allows for rigorous testing and evaluation of the models for safety risks before they are widely deployed. It also provides an opportunity for feedback on potential safety improvements, ensuring that the AI models adhere to ethical guidelines and regulatory standards⁴⁵.
Conclusion
The agreement between OpenAI, Anthropic, and federal authorities marks a significant step towards responsible AI development. The ‘Strawberry’ AI model, with its improved reasoning capabilities, holds the potential to drive substantial progress in various fields. By aligning AI advancements with regulatory and ethical standards, this initiative aims to create a safer and more beneficial AI landscape for all.
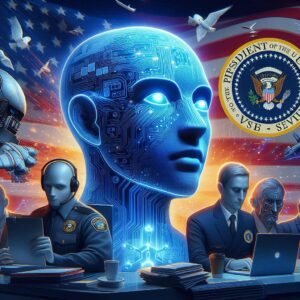
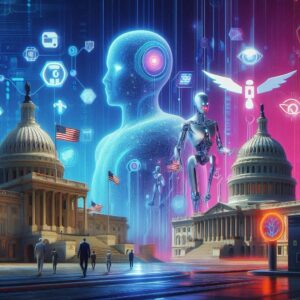
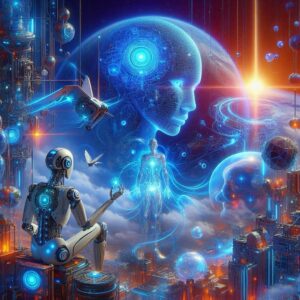
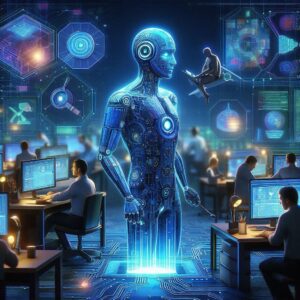
AI technology, while incredibly powerful and beneficial, also comes with several safety risks that need to be carefully managed. Here are some of the key concerns:
1. Bias and Discrimination
AI systems can inadvertently perpetuate or even amplify biases present in the data they are trained on. This can lead to unfair treatment of individuals based on race, gender, age, or other characteristics.
2. Privacy Violations
AI systems often require large amounts of data, which can include sensitive personal information. If not properly managed, this data can be misused or inadequately protected, leading to privacy breaches.
3. Security Threats
AI can be exploited by malicious actors to create sophisticated cyber-attacks, such as deepfakes or automated hacking tools. These threats can compromise the security of individuals, organizations, and even nations.
4. Autonomous Weapons
The development of AI-powered autonomous weapons poses significant ethical and safety concerns. These weapons could potentially make life-and-death decisions without human intervention, raising the risk of unintended consequences.
5. Job Displacement
As AI systems become more capable, there is a risk of significant job displacement in various industries. This can lead to economic instability and increased inequality if not managed properly.
6. Lack of Transparency
Many AI systems, especially those based on deep learning, operate as “black boxes,” meaning their decision-making processes are not easily understood. This lack of transparency can make it difficult to identify and correct errors or biases.
7. Unintended Consequences
AI systems can sometimes behave in unexpected ways, especially if they encounter scenarios that were not anticipated during their development. These unintended consequences can lead to harmful outcomes.
8. Ethical Concerns
The use of AI in areas such as surveillance, predictive policing, and social scoring raises significant ethical questions about the balance between security and individual freedoms.
Mitigation Strategies
To address these risks, several strategies can be employed:
- Robust Testing and Validation: Ensuring AI systems are thoroughly tested in diverse scenarios to identify and mitigate potential risks.
- Regulatory Oversight: Implementing regulations that govern the development and deployment of AI technologies.
- Transparency and Explainability: Developing AI systems that are transparent and whose decision-making processes can be easily understood.
- Ethical Guidelines: Establishing and adhering to ethical guidelines for AI development and use.
- Continuous Monitoring: Regularly monitoring AI systems for any signs of malfunction or misuse.
By proactively addressing these safety risks, we can harness the benefits of AI while minimizing potential harms.
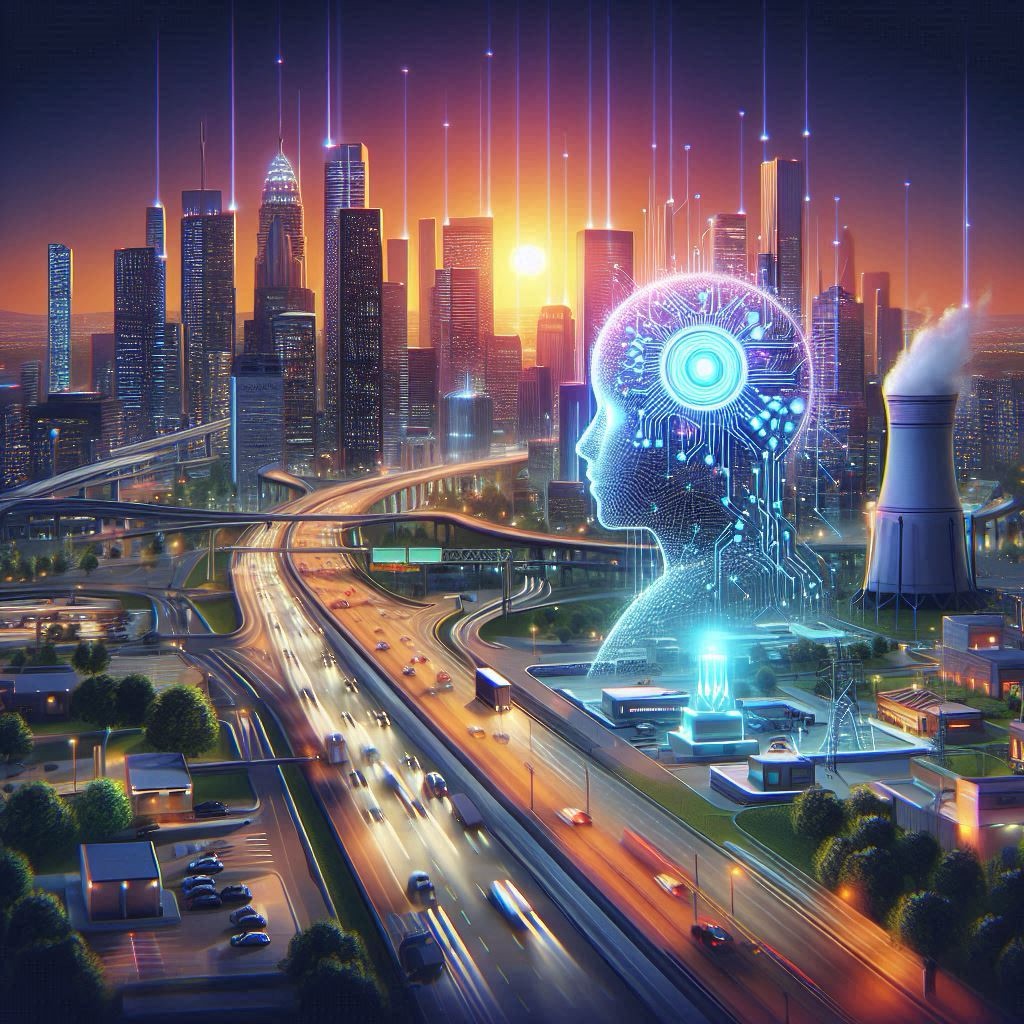
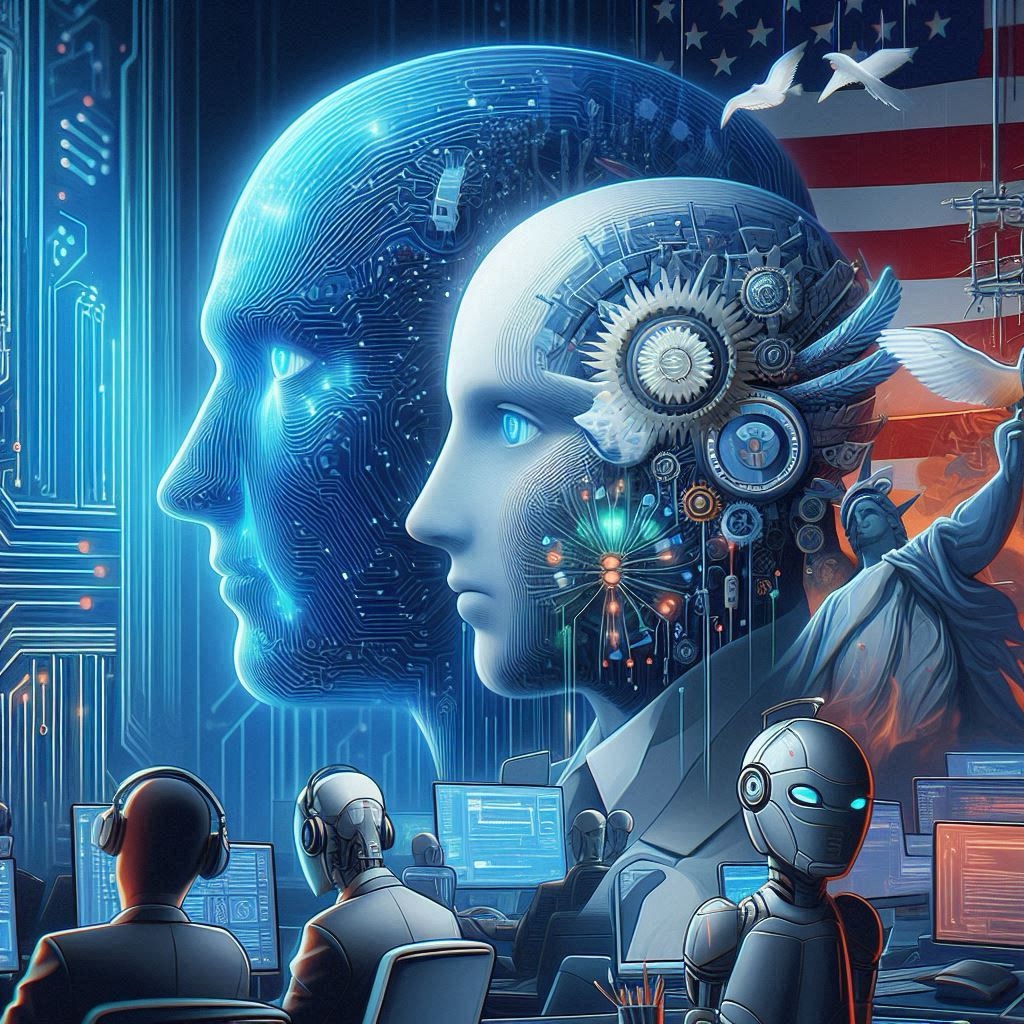
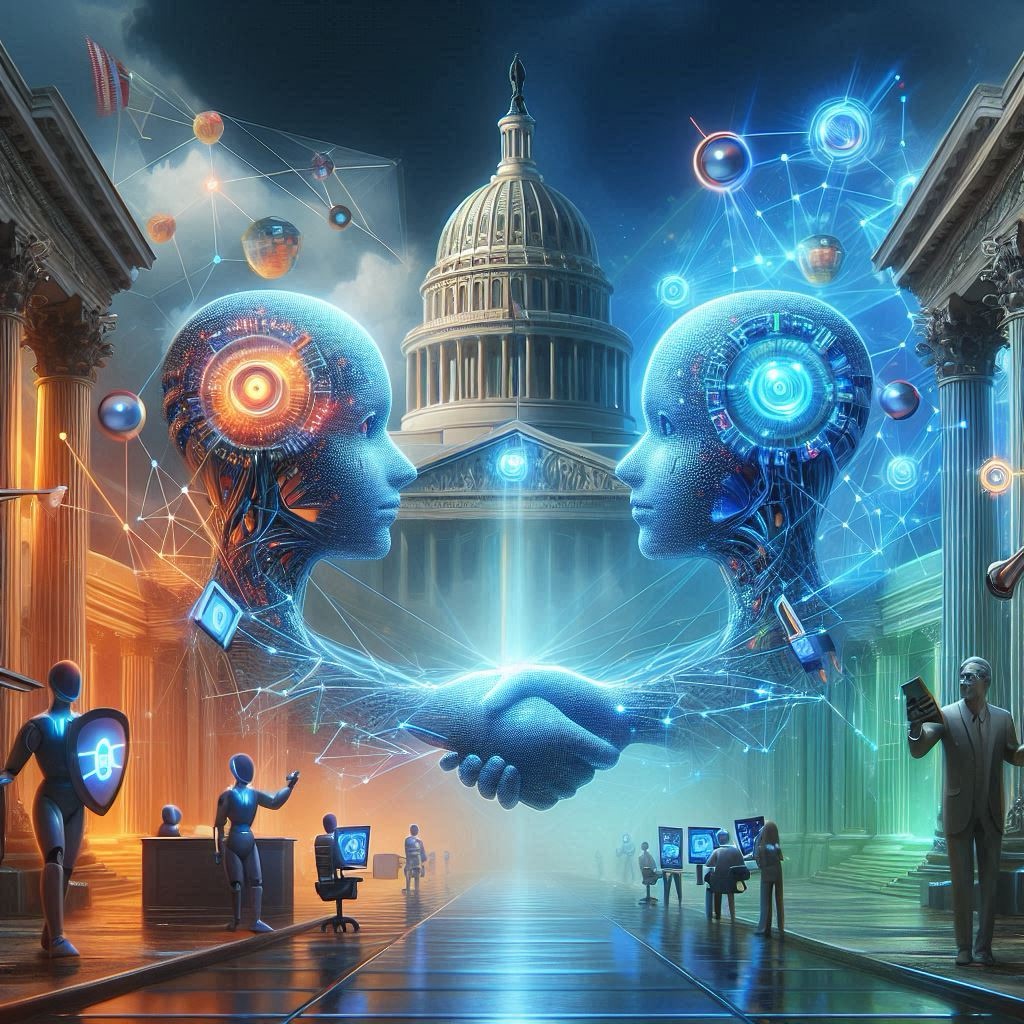
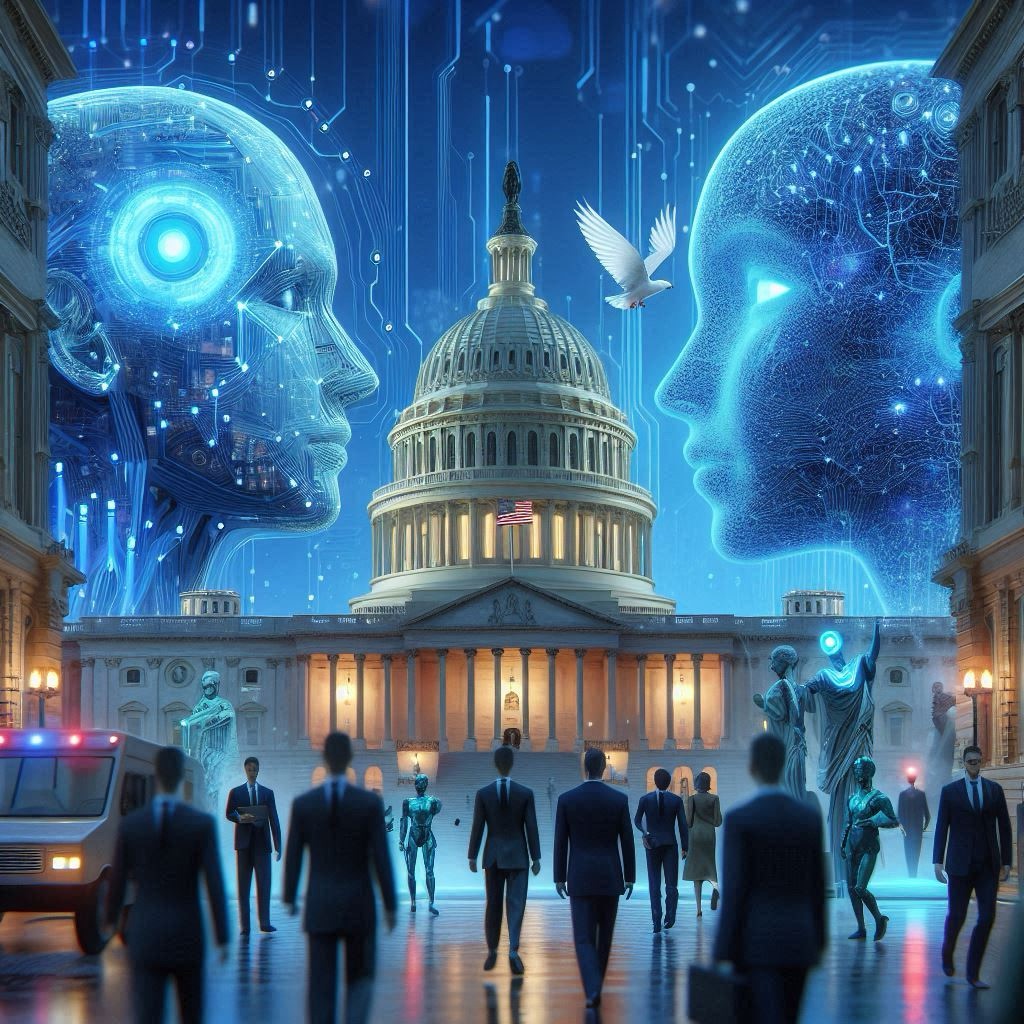
Mitigating bias in AI is crucial for ensuring fairness and equity in AI systems. Here are several strategies to address and reduce bias:
1. Diverse Data Collection
Ensure that the training data is representative of the diverse population it aims to serve. This helps in making more equitable and unbiased decisions⁸.
2. Algorithmic Auditing
Regularly audit algorithms to identify and quantify bias. This involves checking for any disparities in how different groups are treated by the AI system⁸.
3. Blind Taste Tests
Implement “blind taste tests” where the algorithm is denied information suspected of biasing the outcome. This helps in making predictions without being influenced by potentially biased variables¹.
4. Bias-Aware Algorithms
Develop and use algorithms that are specifically designed to detect and mitigate bias. These algorithms can adjust their decision-making processes to account for identified biases⁴.
5. Human Oversight
Incorporate human oversight in the AI decision-making process. This can involve having humans review and validate AI decisions, especially in high-stakes scenarios⁷.
6. Transparency and Explainability
Ensure that AI systems are transparent and their decision-making processes can be easily understood. This helps in identifying and correcting biases more effectively⁶.
7. Ethical Guidelines and Governance
Establish and adhere to ethical guidelines for AI development and use. Implement governance structures to provide ongoing review and oversight of AI systems².
8. Continuous Monitoring and Feedback
Regularly monitor AI systems for any signs of bias and incorporate feedback mechanisms to continuously improve the system³.
By implementing these strategies, we can work towards creating AI systems that are fairer and more equitable.
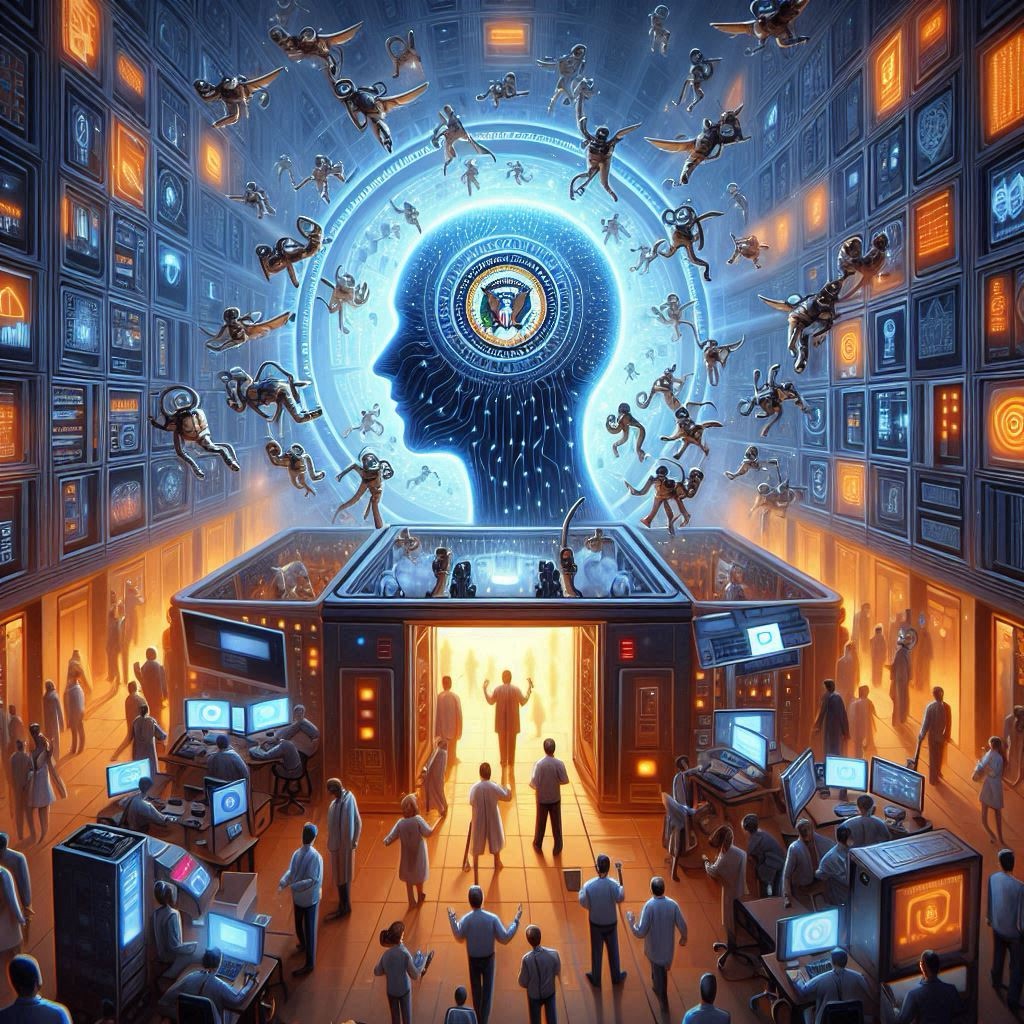
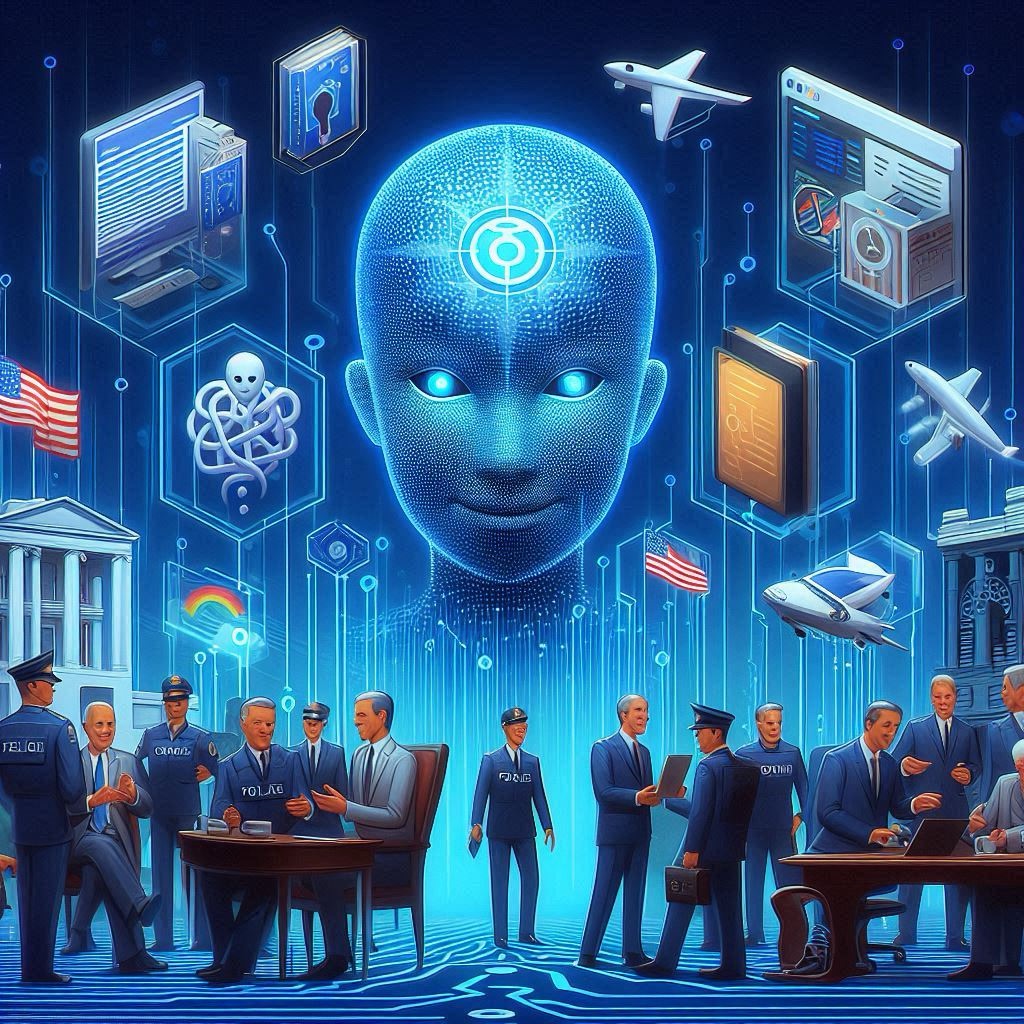
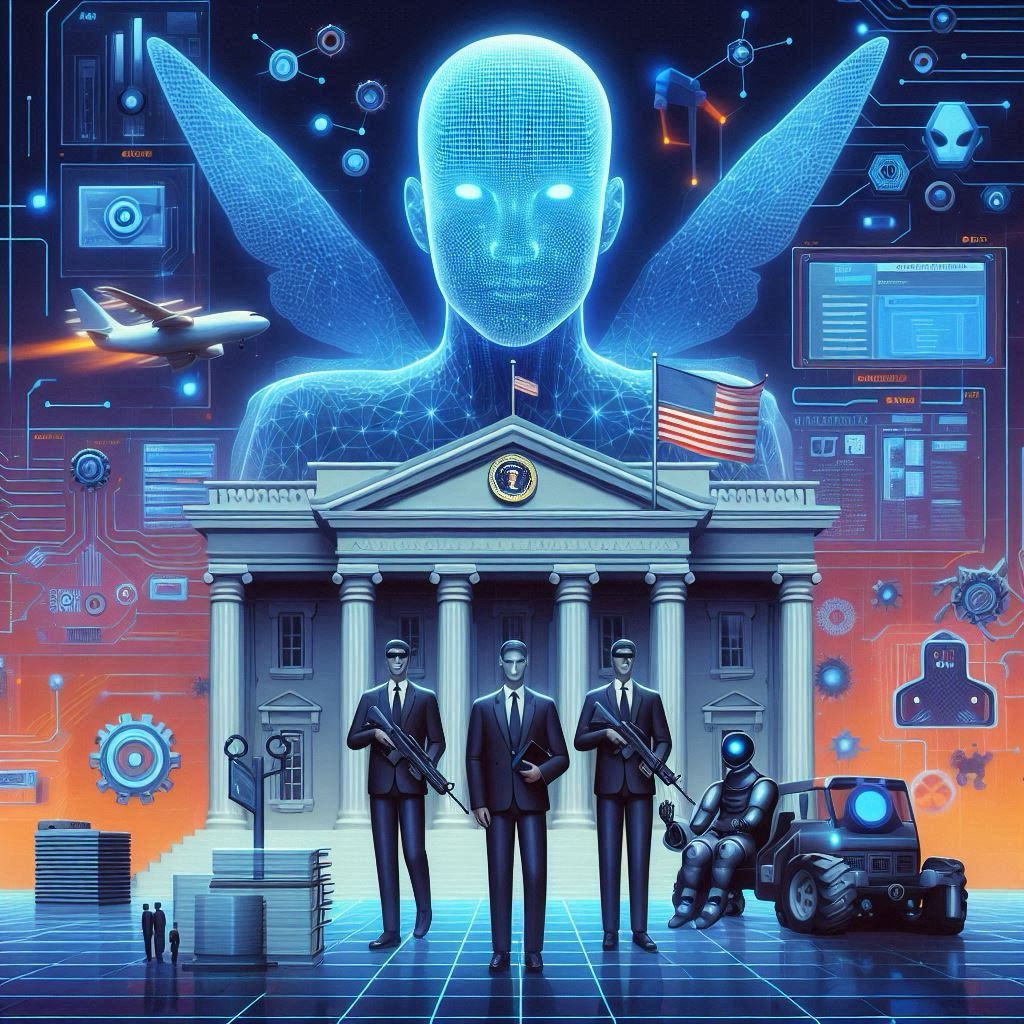
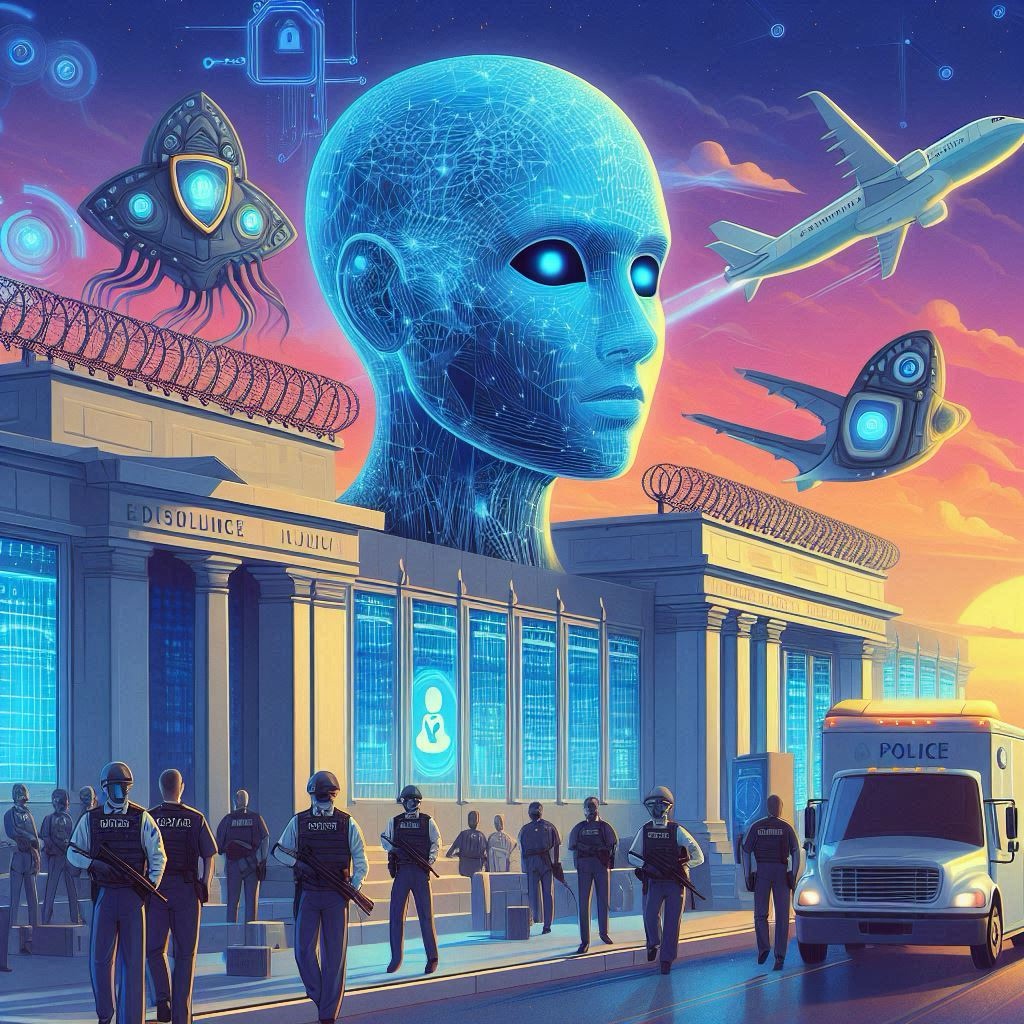
Addressing bias in facial recognition technology is crucial for ensuring fairness and accuracy. Here are several strategies to mitigate bias:
1. Diverse and Representative Datasets
Ensure that the training datasets are diverse and representative of the population. This includes collecting data from various demographic groups to avoid over-representation of any single group¹⁴.
2. Algorithmic Auditing
Regularly audit facial recognition algorithms to identify and correct biases. This involves testing the algorithms on diverse datasets to ensure they perform equally well across different demographic groups¹⁹.
3. Synthetic Data Generation
Use synthetic data to balance datasets. Researchers at NYU Tandon have successfully reduced bias by generating highly diverse and balanced synthetic face datasets⁴.
4. Blind Taste Tests
Implement “blind taste tests” where the algorithm is denied access to potentially biasing information. This helps in making unbiased predictions⁹.
5. Bias-Aware Algorithms
Develop and use algorithms specifically designed to detect and mitigate bias. These algorithms can adjust their decision-making processes to account for identified biases¹.
6. Human Oversight
Incorporate human oversight in the decision-making process. This can involve having humans review and validate the decisions made by facial recognition systems, especially in critical applications⁹.
7. Transparency and Explainability
Ensure that facial recognition systems are transparent and their decision-making processes can be easily understood. This helps in identifying and correcting biases more effectively¹.
8. Ethical Guidelines and Governance
Establish and adhere to ethical guidelines for the development and use of facial recognition technology. Implement governance structures to provide ongoing review and oversight¹.
9. Continuous Monitoring and Feedback
Regularly monitor facial recognition systems for any signs of bias and incorporate feedback mechanisms to continuously improve the system⁹.
By implementing these strategies, we can work towards creating facial recognition systems that are fairer and more equitable.
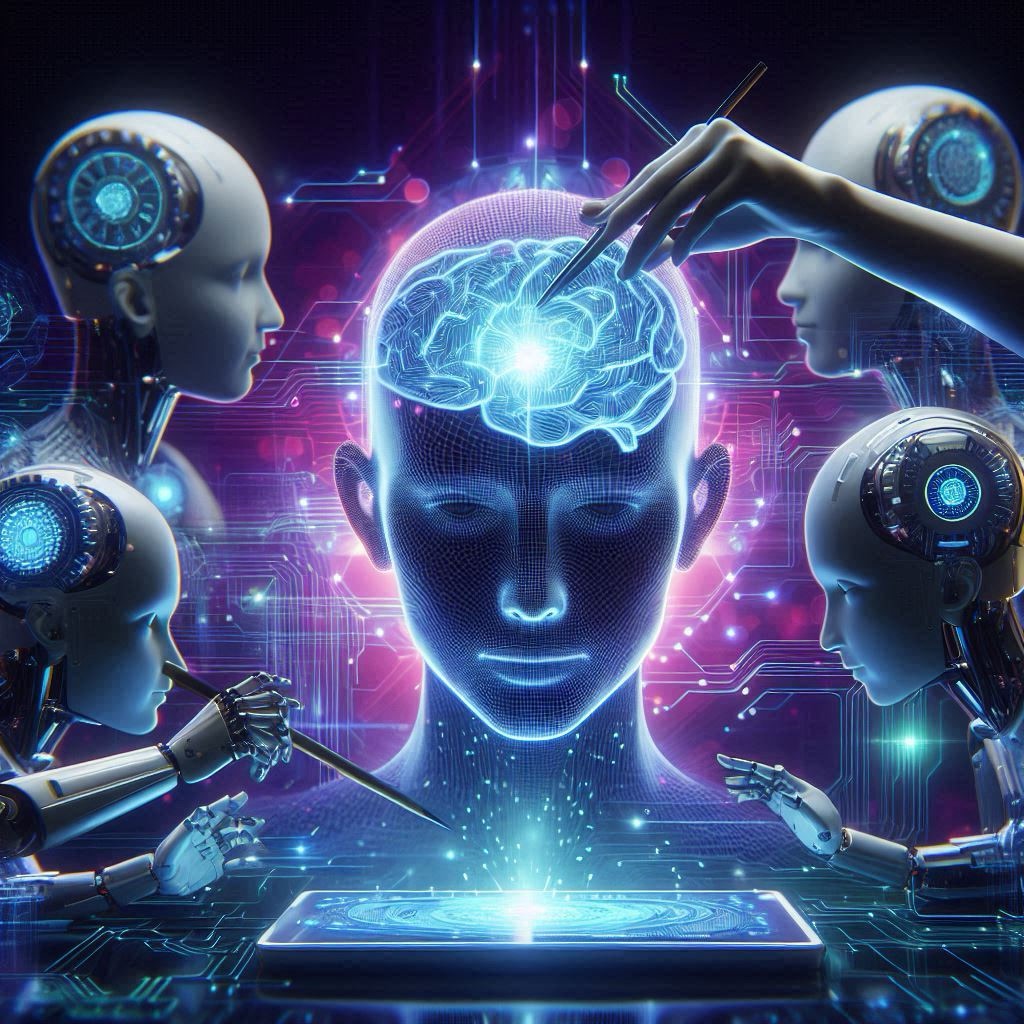
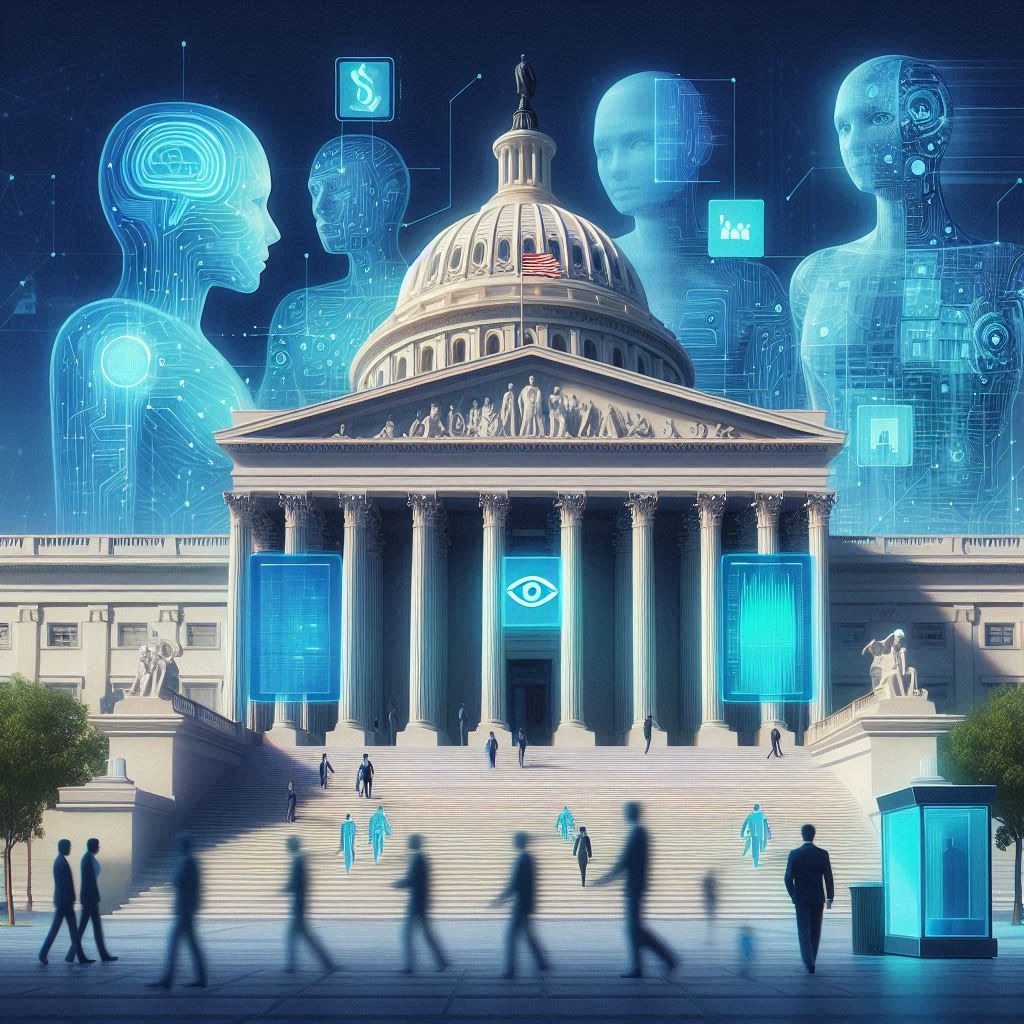
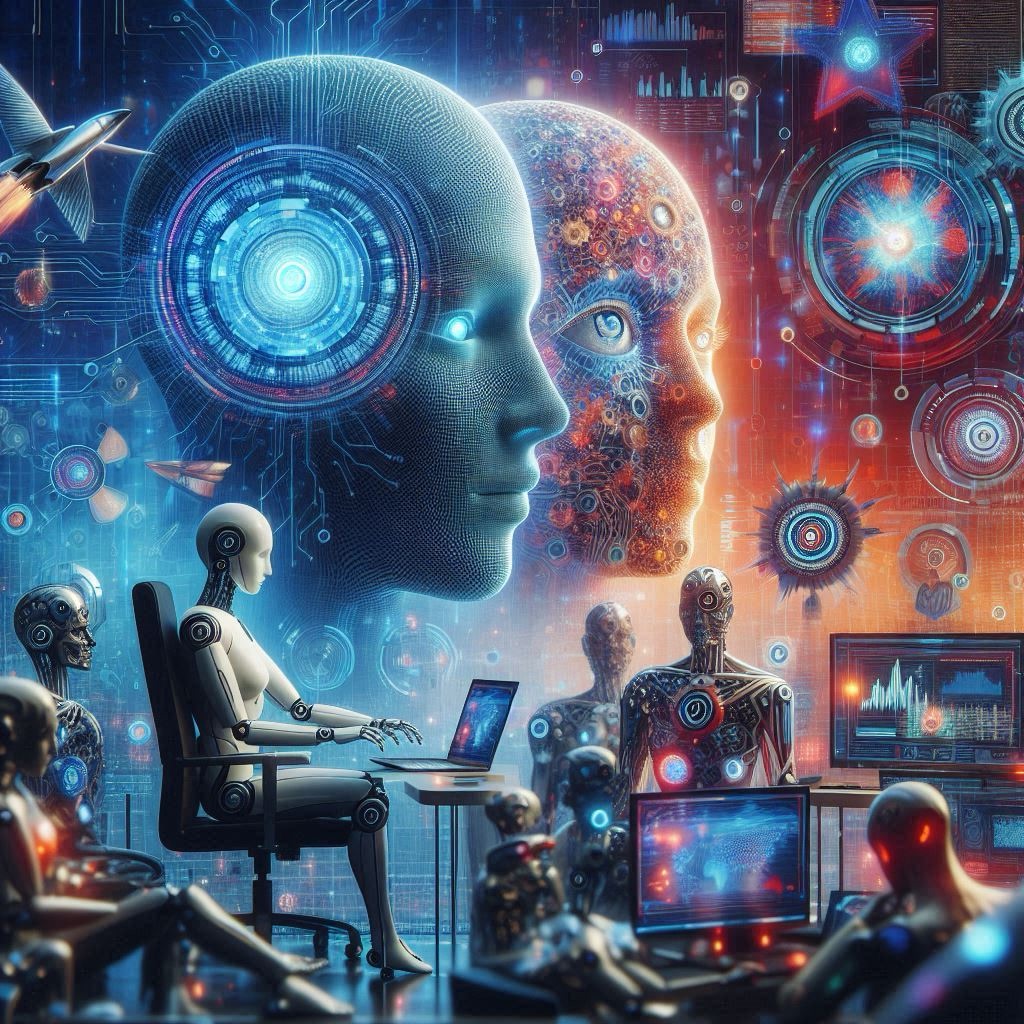
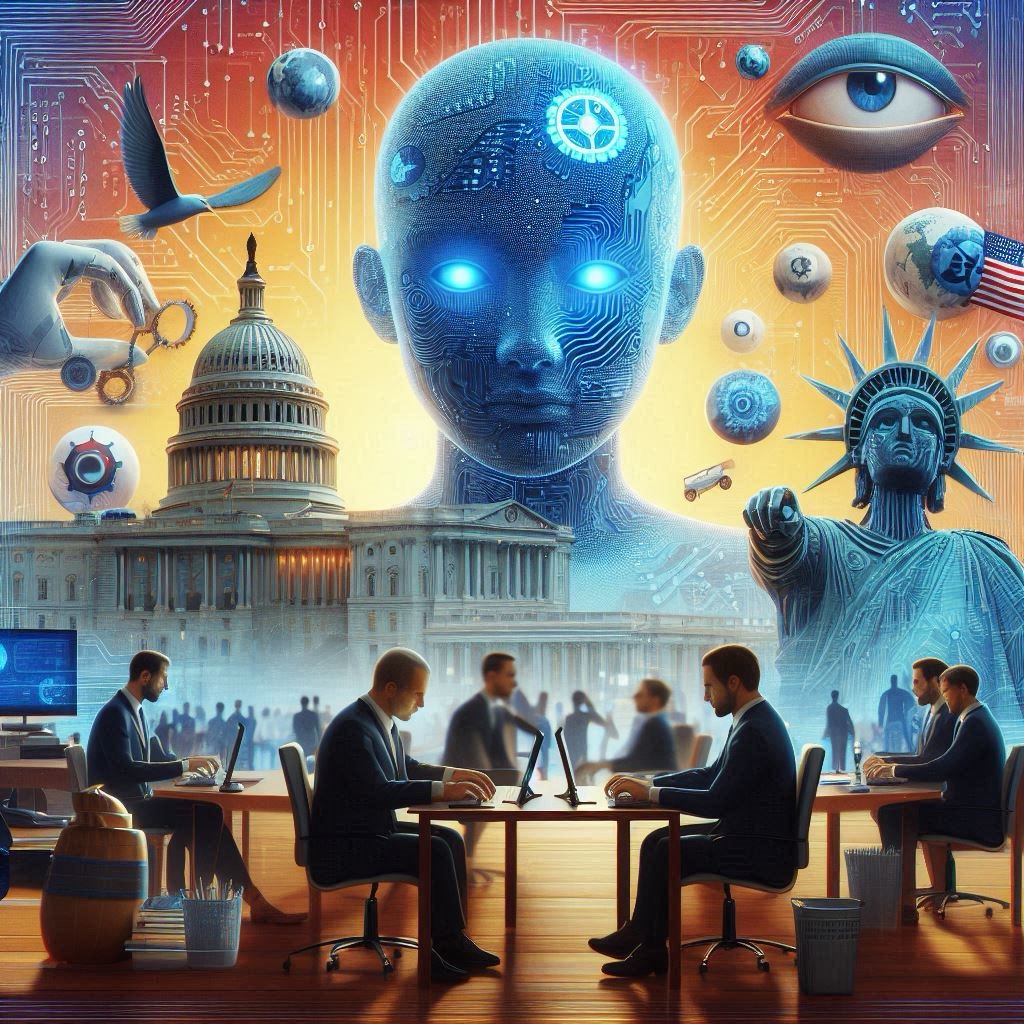
Biased facial recognition technology can have several serious and far-reaching consequences. Here are some of the key issues:
1. Misidentification
Biased facial recognition systems can lead to higher rates of misidentification, particularly among minority groups. This can result in wrongful accusations, arrests, and legal consequences for innocent individuals.
2. Privacy Violations
Inaccurate facial recognition can lead to unwarranted surveillance and tracking of individuals, infringing on their privacy rights. This is particularly concerning in public spaces where people expect a certain level of anonymity.
3. Discrimination
Bias in facial recognition can perpetuate and amplify existing societal biases, leading to discriminatory practices. For example, certain demographic groups might be unfairly targeted or scrutinized more heavily than others.
4. Erosion of Trust
Widespread use of biased facial recognition technology can erode public trust in both the technology and the institutions that use it. This can lead to resistance against the adoption of potentially beneficial AI technologies.
5. Economic and Social Inequality
Biased facial recognition can exacerbate economic and social inequalities by disproportionately affecting marginalized communities. This can limit their access to services, opportunities, and fair treatment.
6. Psychological Impact
Individuals who are frequently misidentified or unfairly targeted by facial recognition systems may experience psychological stress, anxiety, and a sense of injustice.
7. Legal and Ethical Concerns
The use of biased facial recognition technology raises significant legal and ethical questions. It challenges principles of fairness, justice, and equality, and can lead to legal battles and policy changes.
Mitigation Strategies
To address these potential consequences, it is crucial to:
- Ensure diverse and representative training datasets.
- Regularly audit and test algorithms for bias.
- Implement transparency and explainability measures.
- Establish robust ethical guidelines and governance structures.
- Incorporate human oversight and continuous monitoring.
By taking these steps, we can work towards minimizing the negative impacts of biased facial recognition technology and promote its fair and ethical use.